Clearing the Global Environment in R: Complete Guide for Data Scientists
Understand the global environment in r
When work with r, the global environment (likewise call the workspace) stores all the objects you create during a session. These objects include variables, functions, data frames, and other data structures. Over time, specially during lengthy analysis sessions, this environment can become cluttered with unnecessary objects that consume memory and potentially cause naming conflicts.
Clear the global environment is an essential practice for maintain clean, reproducible code and efficient workflow. This guide cover various methods to clear your r environment and best practices for environment management.
Why you should clear your r environment
Before dive into the how-to, let’s understand why clear your environment matters:
-
Memory management
removes objects that consume ram -
Code reproducibility
ensures your code run right without depend on ppre-existingobjects -
Prevents variable conflicts
avoids confusion from old variable definitions -
Clean slate for new analysis
provides a fresh start for different projects -
Debug assistance
helps isolate issues by eliminate variables from previous runs
Methods to clear the global environment in r
Method 1: use the rm () function
The virtually common approach to clear objects from your environment is use the
Rm ()
Function, which remove specify objects:
- remove all objects from the environment rm(list = ls ()
This command work by:
-
Ls ()
Lists all objects in the current environment -
List = ls ()
Pass this list to the
Rm ()
Function -
Rm ()
Remove all objects in the list
If you want to keep certain objects while remove others, you can use pattern matching:
- remove all objects except those start with" keep _ " m(list = ls()[!grgrep^ke^ keep", ls ( (] ]
Method 2: user studio’s interface
If your ususer studioyou can clear the environment through the graphical interface:
- Look for the broom icon in the environment pane
- Click the icon to clear all objects
- Instead, use the dropdown menu following to the broom icon for more options
This method is equivalent to run
Rm(list = ls ()
But provide a convenient one click solution.
Method 3: use cat(“14” ) or cCtrll to clear the console
While this doesn’t clear the environment, it’s useful to know how to clear the console output:
- clear the console cat("14" )
Instead, you can use the keyboard shortcut Ctrl+l (cmd+l on mMac) Remember that this entirely clear the console display, not the actual environment objects.
Method 4: use the assign () function
A less common but sometimes useful approach is to create a new empty environment:
- create a new environment and assign it to.global env assign(".Globalenvv ", new.env( ), never = as.environment("package: base" )
This method is more advanced and mostly not recommend unless you have specific needs.
Method 5: restart r
The about thorough approach is to restart your r session all:
- In studio: session → restart r ((r ctCtrlhift+f10 ))
-
In r console:
Q ()
To quit, so restart r
Restart r not but clear the environment but likewise reset load packages and other session information.
Clearing specific types of objects
Remove only certain objects
You can selectively remove objects base on their names:
- remove specific objects by name rm(variable1, variable2, data_frame1)
Or use patterns to match object names:
- remove all object start with" temp _ " m(list = ls(pattern = "" e^ temp""
Remove objects by type
You can too remove objects base on their class or type:
- function to remove all data frames rm_data frame function (( { {jobis envirneverobaglobal en)remove < sa y(supplyfobision(x ) is.d)a.frame(get(x ) rm(li) = objs[to_robise ], envir].glnevernv global en)
Manage environments in r projects
Use r projects for environment isolation
Studio projects provide a way to keep work organize in separate environments:
- Create a new project: file → new project
- Each project maintain its own workspace and work directory
- Switch between projects mechanically change environments
This approach help maintain separation between different analyses and prevent variable conflicts.
Use.profile for automatic environment clearing
You can set up your r session to mechanically clear the environment when start:
- add to your.profile file.first < unction ( )( if{ int(active ( ) { (t("{cleare Clairement for a fresh start... n " ) rm(l" = ls(envir =.glnevernv global en).glnevernv global en)
This ensures you invariably start with a clean environment.
Best practices for environment management in r
Use script base workflows
Preferably than rely on objects in your environment, develop the habit of create reproducible scripts:
-
Start scripts with environment clearing:
Rm(list = ls ()
- Load all necessary packages at the beginning
- Include all data import steps in your script
- Avoid save the workspace between sessions
This approach ensure your analysis can be reproduced from scratch.
Set studio options for better environment management
Configure studio to support clean environment practices:
- Go to tools → global options
-
Under general → basic, set” save workspace to.rdataon exit “” ” ” er ”
” - Uncheck” restore.rdatainto workspace at startup “”
These settings prevent carry over objects between sessions, encourage better code practices.
Use environment snapshots
Before clear your environment, you might want to save its state:
- save current environment to a file save.image(file =" environment_backup. rRdata" - tardy, restore ifif youeed load("environment_backupRdata" " )
This allows you to clear your environment while maintain the ability to restore itif needed need.
Advanced environment management techniques
Work with multiple environments
R allow you to create and manage multiple environments:
- create a new environment my_env < new.env ( (- add objects to this environment assign("x ", 10, envnevermy_env ) )access object from this environment my_env$envoyr get("x ", envinevery_env ))
This approach helps organize related objects and prevent namespace pollution.
Use packages like Pac-Man for environment management
The
Pac-Man
Package provide useful functions for manage environments:
- install if not already install if (!RequireePac-Ma) ) install.packages(Pac-Ma" " ) - load packages and clear environment in one stepPac-Mann::p_loadulyyr, ggplot)) Pac-Man::p_clear( ) - clear the global environment
Environment management in shiny applications
When develop shiny apps, environment management become flush more critical:
- in server. R or app. R function(input, output, session) {{ use rereactive valueslternatively of global variables values < eareactive values(- initialize variables within the reactive context values$data < l - cleared specific reactive values when need obserobserve eventt$reset, { va{es$data < nu } ) }}}
Troubleshoot common environment issues
Object not being removed
If
Rm(list = ls ()
Doesn’t seem to remove all objects, check for:
- Hidden objects (names start with “. ” )
- Objects in different environments
- Locked bindings
To remove hidden objects as easily:
Rm(list = ls(all.names = true)
Memory not being release
Sometimes clearing objects doesn’t instantly free memory. To force garbage collection:
Rm(list = ls () gGC((
This explicitly tell r to reclaim unused memory.

Source: community.rstudio.com
Package object persist
Objects from packages aren’t in the global environment, hence
Rm(list = ls ()
Won’t will affect them. To unload a package:
Detach("package: package name ", unload = tru))
For a complete reset, restart r is the nigh reliable option.
Conclusion
Maintain a clean global environment in r is essential for efficient and reproducible data analysis. Whether you prefer the simple
Rm(list = ls ()
Approach, studio’s interface, or more advanced environment management techniques, regular clearing of your workspace will improve your r programming experience.
By will incorporate these practices into your workflow, you will avoid common pitfalls like memory issues, variable conflicts, and nonnon-reproduciblede. Remember that the goal isn’t simply to clear your environment occasionally, but to develop a systematic approach to environment management that support your data science work.

Source: darelovisions.weebly.com
For complex projects, consider use r projects, custom environments, and script base workflows to maintain organization and reproducibility. With these tools and techniques, you’ll be easily will equip to will manage your r environment efficaciously.
MORE FROM gowithdeal.com
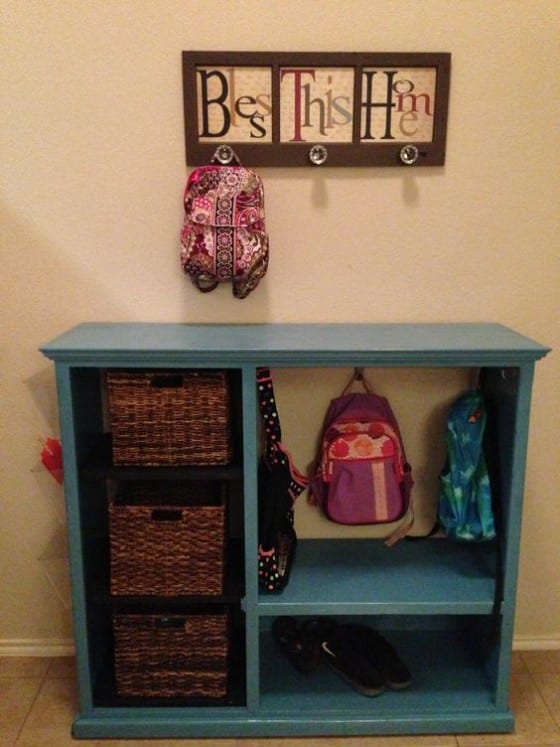
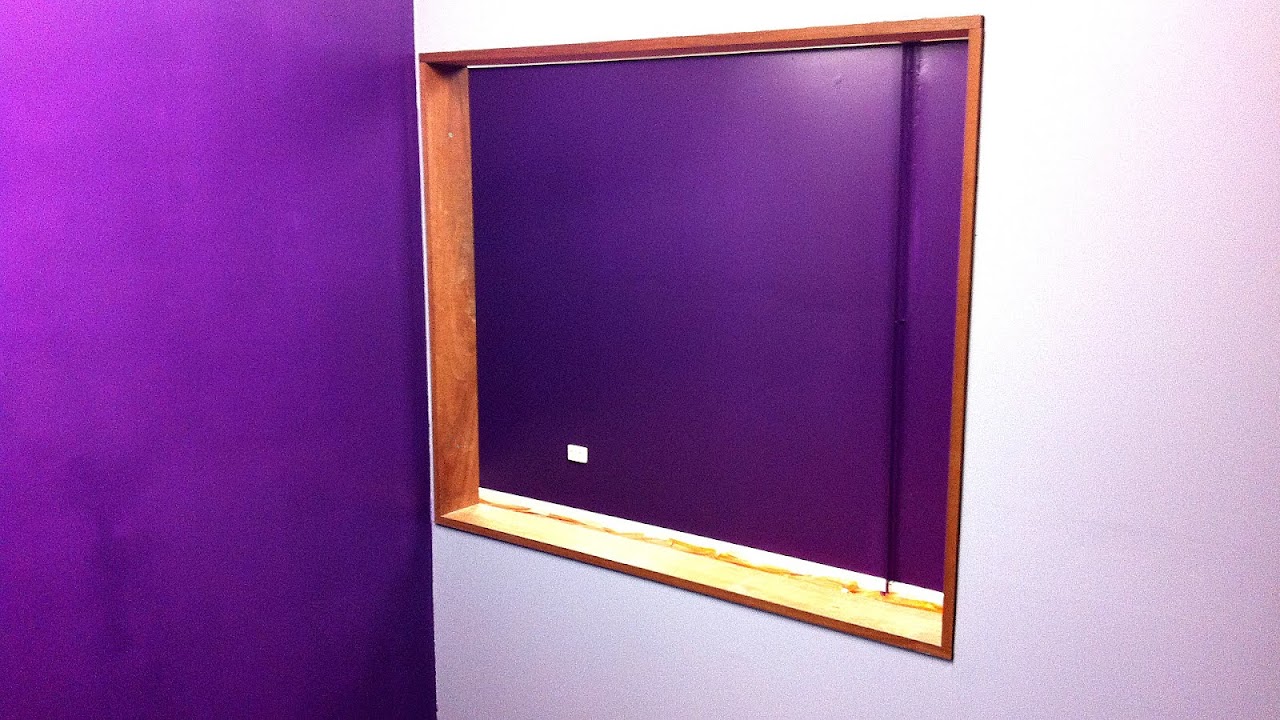
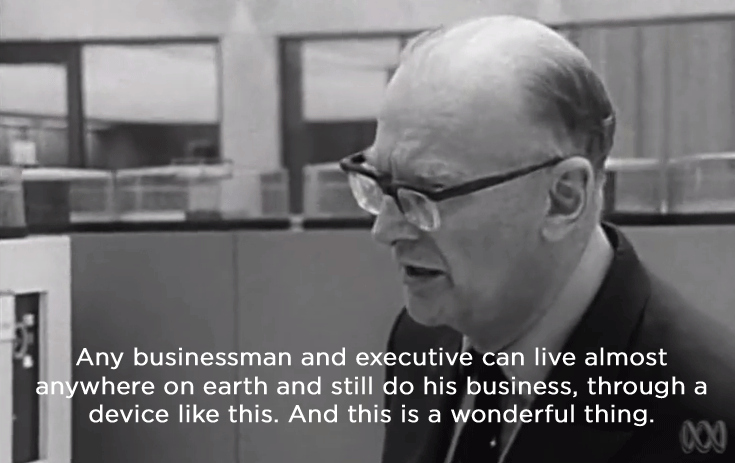
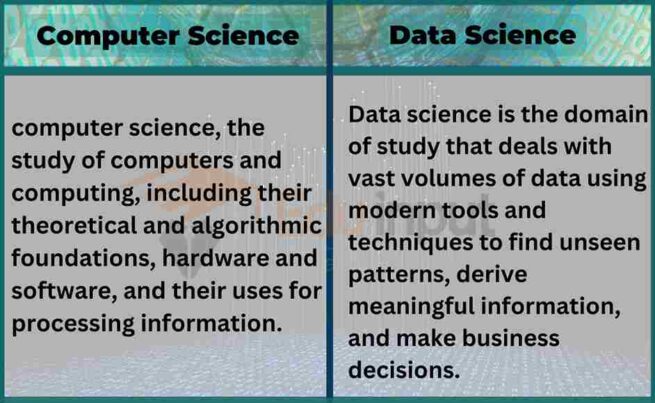
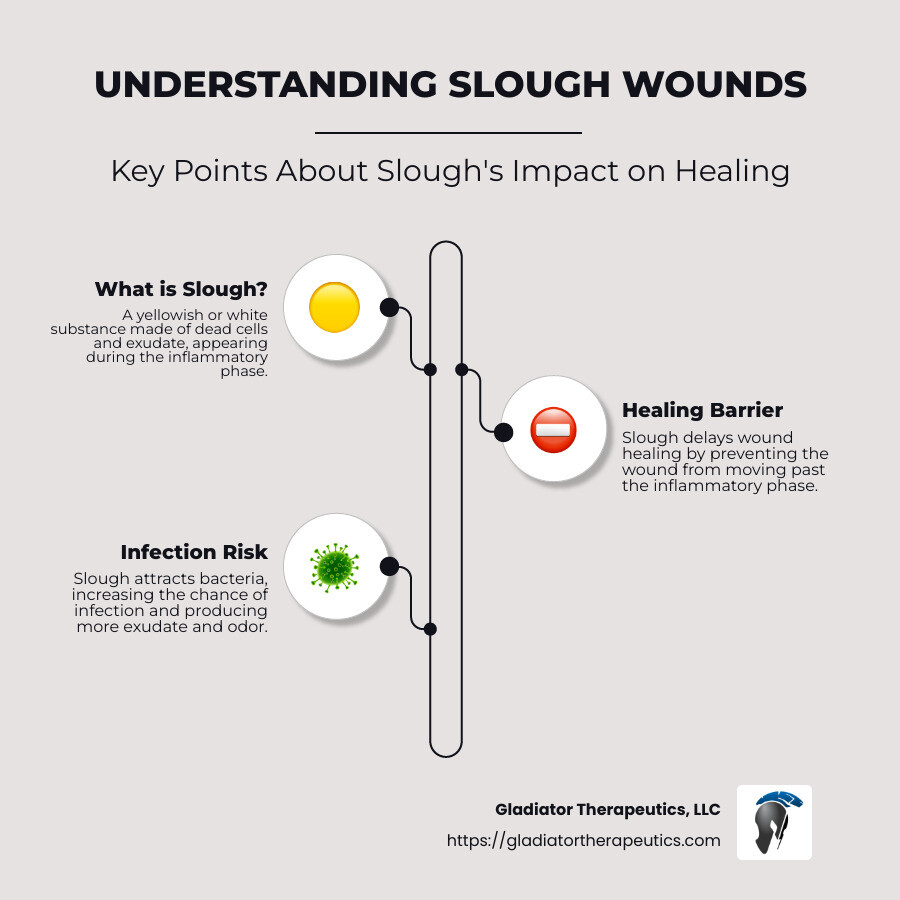
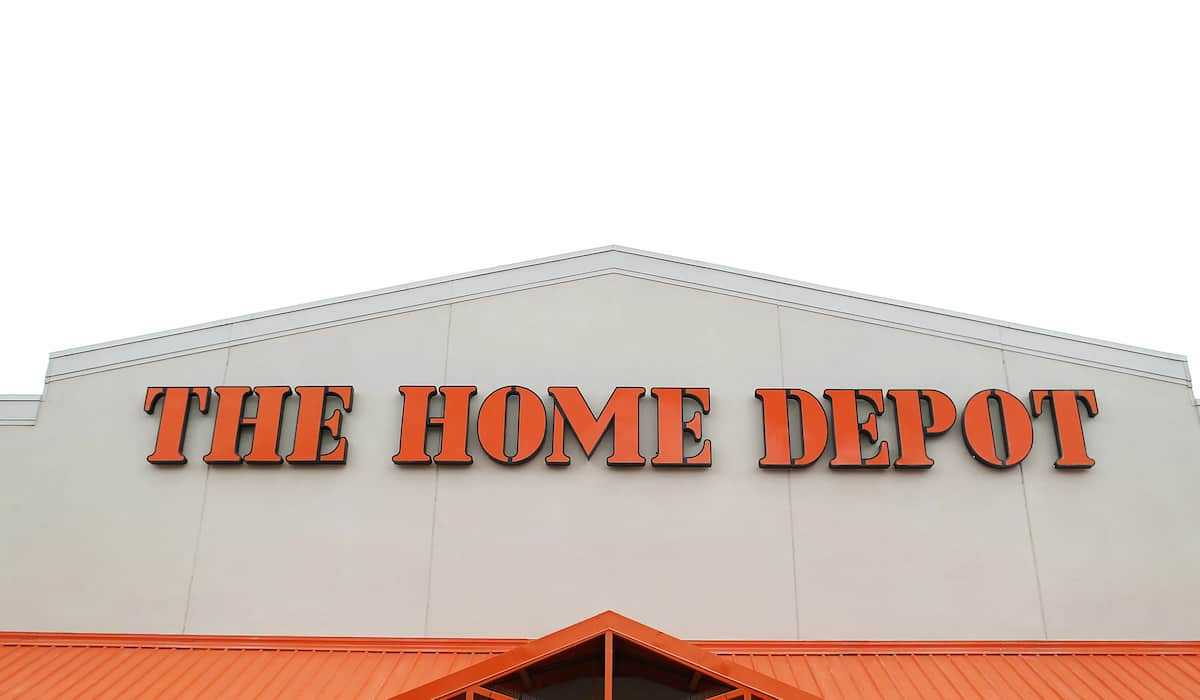
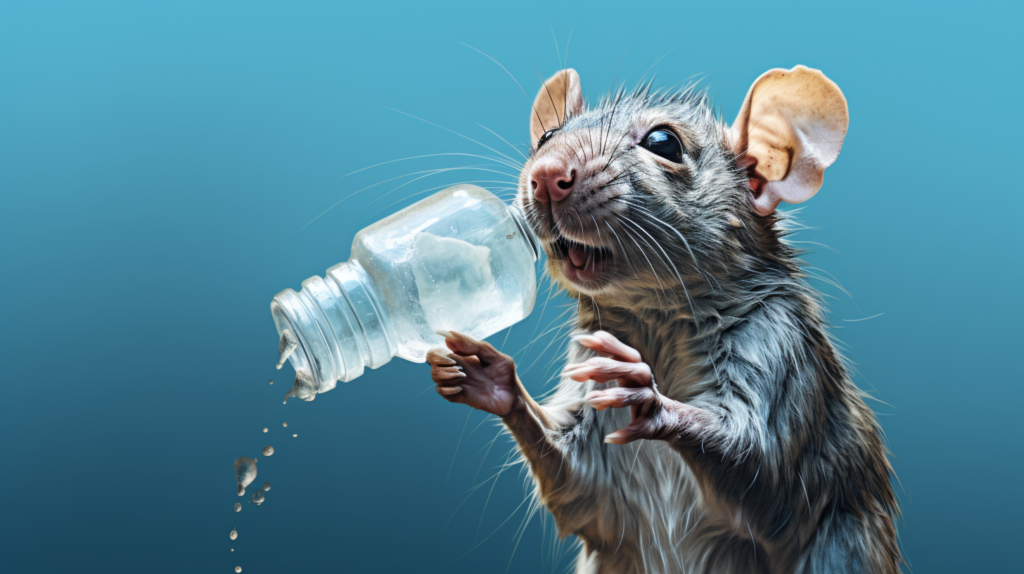
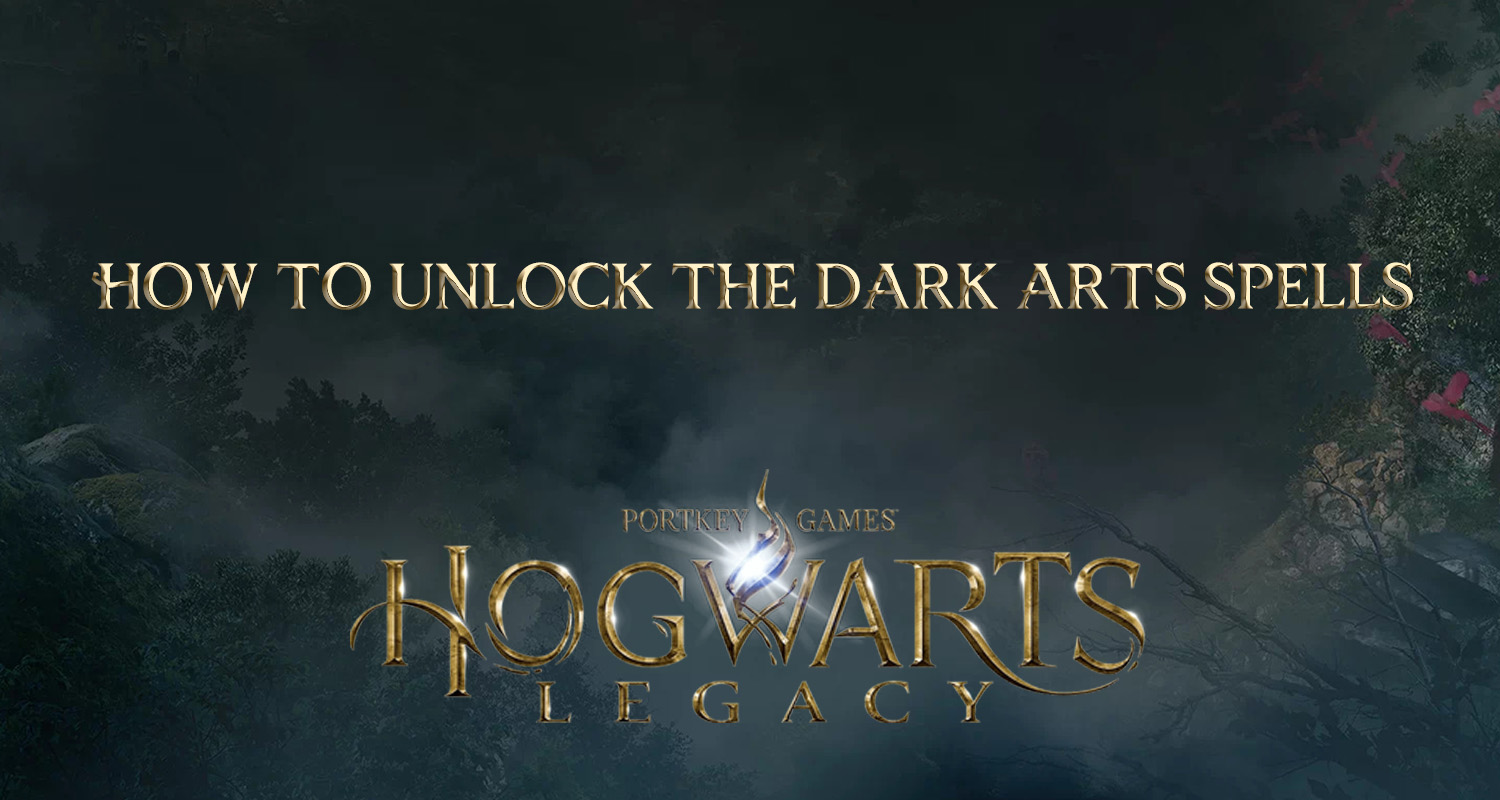
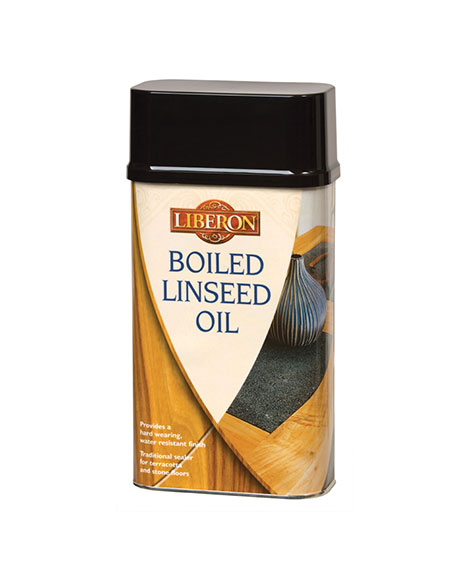
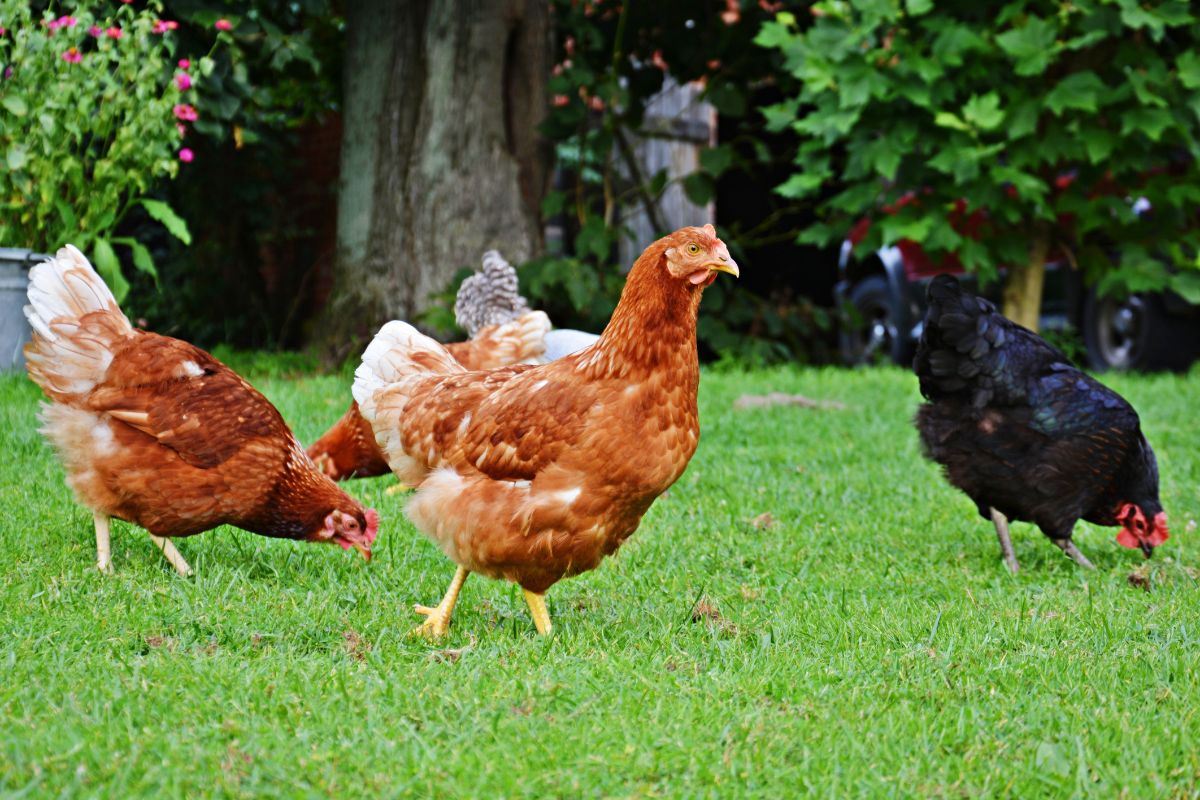
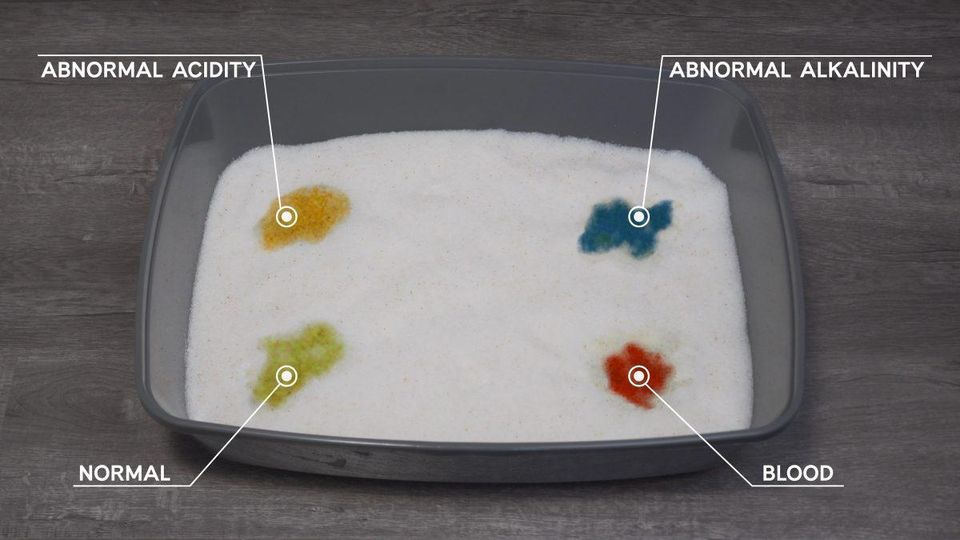
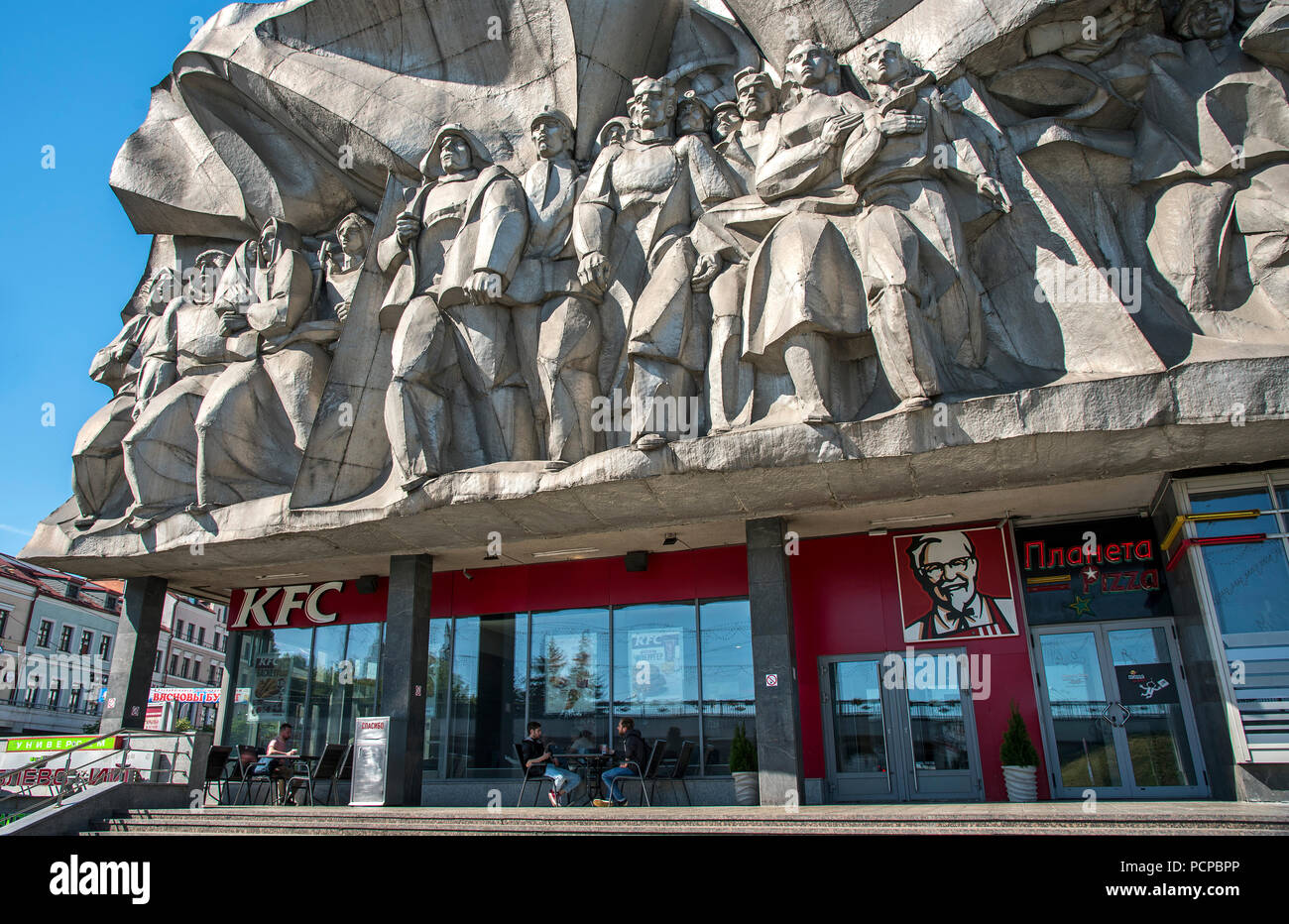